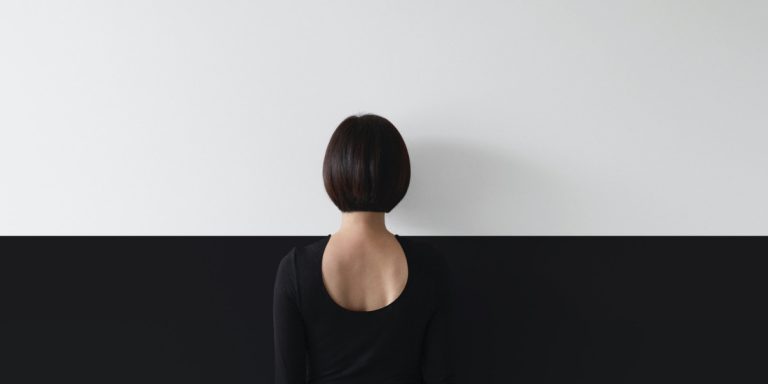
Offered by Intel
AI and privacy needn’t be mutually unique. After a decade in the labs, homomorphic encryption (HE) is rising as a high manner to assist defend data privacy in machine studying (ML) and cloud computing. It’s a well timed breakthrough: Data from ML is doubling yearly. At the similar time, concern about associated data privacy and security is rising amongst trade, professionals and the public.
“It doesn’t must be a zero-sum sport,” says Casimir Wierzynski, senior director, workplace of the CTO, AI Merchandise Group at Intel. HE permits AI computation on encrypted data, enabling data scientists and researchers to achieve priceless insights with out decrypting the underlying data or fashions. That is notably vital for delicate medical, monetary, and buyer data.

Above: Casimir Wierzynski, Senior Director, Workplace of the CTO, AI Merchandise Group at Intel
Wierzynski leads Intel’s privacy preserving machine studying efforts, together with HE work and in growing trade requirements for the expertise’s use. On this interview, he talks about why HE is required, its place as a constructing block of improved privacy, and how the breakthrough expertise helps create new enterprise alternatives and data bridges to beforehand “untrusted companions.”
What and why
Q: What’s does “homomorphic” imply?
In Greek, homo is the similar, morphic is the form. So it captures the thought that in the event you do encryption in the proper manner, you may remodel unusual numbers into encrypted numbers, then do the similar computations you’ll do with common numbers. No matter you do on this encrypted area has the similar form as what you’re doing in the common area. Once you convey your outcomes again, you decrypt again to unusual numbers, and you’ll get the reply you wished.
Q: What large downside is the expertise is fixing?
We stay in superb occasions, when we now have all these new AI merchandise and providers, like with the ability to unlock your cellphone along with your face, or programs that allow radiologists to detect ailments at earlier levels. These merchandise rely on machine studying programs, that are fed and formed by data that are very delicate and private. It’s vital for us as an trade — and I might argue as a society — to determine how we will maintain unlocking all the energy of AI whereas nonetheless serving to defend the privacy of the underlying data. That’s the overarching downside.
Q: Why is HE creating buzz now?
The approach itself has been round for greater than 20 years as a theoretical assemble. The criticism has been, okay, you may function on encrypted data, but it surely takes you 1,000,000 occasions longer than utilizing common data. It was a tutorial curiosity. However in the final 5 years, and particularly the final two years, there have been large advances in the efficiency of those methods. We’re not speaking a couple of issue of 1,000,000 anymore. It’s extra like an element of 10 to 100.
Q: Definitely, there’s no scarcity of data privacy and safety applied sciences…
We’ve all gotten good about encrypting data at relaxation, when it’s on our arduous drives, or sending data again and forth over encrypted channels. However if you’re coping with ML and AI, in some unspecified time in the future these data must be operated on. That you must do some math on these data. And to do that it’s essential to decrypt them. Whilst you’re utilizing the data, the data are decrypted, and that creates a possible problem. We work to supply better safety on each these fronts, anonymization and encryption.

Above: Easy mannequin of safe inference with HE.
Q: In ML with a number of companions, belief is an enormous problem. How does HE assist right here?
Everytime you’re coping with digital property, you’ve this phenomenon: Once you share a digital asset with one other social gathering, it’s fully equal to giving it to them, then trusting they’re not going to do one thing unintended with it.
Now add the reality that ML is essentially an operation that entails a number of stakeholders. For instance, one entity owns the coaching data. One other entity owns data they need to do some inference on. They need to use ML service offered by a 3rd entity. Additional, they to make use of an ML mannequin owned by one other social gathering. And so they need to run all this on infrastructure from some provide chain.
With all these completely different events, and due to the nature of digital data, all should belief one another in a fancy manner. That is turning into tougher and tougher to handle.
HE in motion
Q: Are you able to give an instance of homomorphic encryption at work?
Say you’ve a hospital doing a scan on a affected person, working with a distant radiology service. The hospital encrypts the scan and sends it over to the radiologist. The radiologist does all the processing in the encrypted area, in order that they by no means see the underlying data. The reply comes again, which can also be encrypted, and lastly the hospital decrypts it to be taught the analysis.
Q: In the instance above, the place does HE truly occur? At the scanner?
It may stay in a number of locations. There are two main elements: encryption and decryption. However then there’s additionally the precise processing of the encrypted data. Encryption usually occurs the place the delicate data are first captured, for instance, in a digital camera or edge machine. Processing encrypted data occurs wherever the AI system must function on delicate data, usually in a data middle. And at last, decryption occurs solely at the level the place it’s essential to reveal the outcomes to a trusted social gathering.
Highly effective with different rising techs
Q: Once you communicate and write on HE, you additionally speak about adjoining applied sciences. Please clarify briefly the roles these different constructing blocks play in preserving privacy.
There are a number of very promising and quickly growing applied sciences that use tips from cryptography and statistics to do operations on data that appear magical.
All these applied sciences may be additional accelerated and enhanced by {hardware} security measures known as trusted execution environments, resembling Intel SGX.
New bridges to companions in AI and ML
Q: So what alternatives are created when these applied sciences are mixed?
One in every of the questions we’ve been asking at Intel is, what would occur in the event you may allow ML in these multi-stakeholder operations between events that don’t essentially belief one another, like we described earlier? What would that allow?
You can have banks that usually are rivals. However they might resolve it’s of their curiosity to cooperate round sure dangers that all of them face in frequent, like fraud and cash laundering. They might pool their data to collectively construct anti-money laundering fashions whereas retaining their delicate buyer data non-public.
One other instance is in the retail sector. Retailers need to make the most out of the data that they’ve collected on consumers, to allow them to personalize sure experiences. What if there have been a option to allow that and nonetheless present quantifiable protections round the privacy of that data?
New enterprise alternatives
Q: Are there new income fashions and alternatives being created?
One factor that’s thrilling about this space is that you begin to allow new enterprise fashions round data that would have beforehand been inconceivable. For instance, (HE) generally is a option to monetize data whereas serving to preserve security. At the similar time, it addresses one in all the largest issues in ML, specifically, entry to giant, numerous data units for constructing fashions. So you may think about an entire ecosystem that brings collectively individuals who maintain data with individuals who want data. And really importantly, it’s all achieved in a manner that preserves security and privacy of the data. That’s an thrilling chance.
Q: How superior is that idea in implementation? Every other or many real-life cases like that?
I might say it’s past concept, and in early levels of business deployment.
Data is turning into a a lot greater asset class than ever earlier than, generally in stunning methods. For instance, in company chapter instances, collectors can find yourself proudly owning giant data units unrelated to banking in any manner. They’re simply leftovers of a mortgage that went bitter. In order that they’re trying for a option to monetize these property and present them to data scientists who search extra coaching data to make their AI programs extra dependable, all whereas retaining the underlying data non-public and safe.
Or think about a bunch of hospitals that have affected person data. For varied causes, they will’t share it. However they know if they may, and may get legal professionals in the room to hammer out an settlement, they’d doubtlessly have the ability to collectively construct a mannequin with rather more statistical energy than one any may construct individually. Utilizing privacy-preserving ML methods, they will primarily kind a consortium and say: “In alternate for all of us proudly owning this improved mannequin that will enhance our sufferers’ outcomes, we’ll be part of this consortium, and we will nonetheless maintain all of our affected person data non-public and safe.”
Key function of builders
Q: The place do builders slot in?
As it’s now, in the event you’re an AI data scientist and you need to construct a machine studying mannequin that operates on encrypted data, it’s a must to be some magical individual, concurrently an knowledgeable in software program engineering and data science and post-quantum cryptography.
One in every of the main efforts that my crew has been working on is making these applied sciences rather more accessible and performant for the data science and developer communities so that they will scale up. This can be a precedence for Intel. Immediately we’re providing instruments like The Intel HE transformer for nGraph, which is a Homomorphic Encryption (HE) backend to Intel’s graph compiler for Synthetic Neural Networks.

Above: The nGraph-HE software program stack helps the SEAL encryption library and underlying cryptosystems.
Requirements and Intel
Q: Some individuals will ask: Why is Intel energetic right here? The reply is…
For starters, homomorphic encryption may be very compute-intensive. That is an space the place Intel can actually shine when it comes to constructing optimized silicon to deal with this essentially new manner of computing.
However extra broadly, these examples from well being care, retail, and finance — sectors representing a couple of quarter of GDP in the United States. These are very economically vital issues. At Intel, we’re obsessive about serving to prospects resolve their issues round data. And privacy is at the coronary heart of any data-centric enterprise.
We’re in a singular place, as a result of Intel works intently with hyperscale, data-centric customers of {hardware} who’re constructing all types of thrilling AI purposes. At the similar time, we’re a impartial social gathering with respect to data. To make these applied sciences carry out at scale goes to require the sorts of complicated software program and {hardware} co-design that Intel is uniquely positioned to supply. We get to collaborate actively with an enchanting vary of gamers throughout trade.

Above: The 2019 HomoEncyption.org requirements group assembly gathered high non-public and public sector individuals from round the globe in Santa Clara, CA.
Q: Intel has taken a management function in growing HE requirements. Why are they vital? Standing?
As with all crypto scheme, individuals will use it when there’s interoperability and belief in the underlying math and expertise. We acknowledge that to essentially scale up, to convey all the homomorphic encryption goodness to the world, we have to have requirements round it.
As curiosity in privacy preserving strategies for machine studying grows, it’s important for requirements to be debated and agreed upon by the group, spanning enterprise, authorities, and academia. So in August, Intel co-hosted an trade gathering of people from Microsoft, IBM, Google, and 15 different corporations on this house.
We’ve recognized many factors of broad settlement. Now we’re making an attempt to determine the proper method to convey it to requirements our bodies, like ISO, IEEE, ITU, and others. All of them have completely different constructions and timelines. We’re strategizing on how finest to maneuver that ahead.
Closing phrase
Q: Any ideas you’d like to depart ringing in individuals’s ears?
Privacy and security applied sciences for machine studying like homomorphic encryption are prepared for prime time. These should not tutorial workout routines anymore. These are actual market-ready concepts. The day is coming when the thought of doing machine studying on another person’s uncooked data will appear fairly unusual. We’re at the begin of a new and thrilling period the place machine studying will allow us to discover new alternatives not like something earlier than.
Sponsored articles are content material produced by an organization that is both paying for the publish or has a enterprise relationship with VentureBeat, and they’re all the time clearly marked. Content material produced by our editorial crew is rarely influenced by advertisers or sponsors in any manner. For extra data, contact gross sales@venturebeat.com.